How Google's AI powers responsive search ads: a technical guide for advertisers
Detailed analysis of Google's AI-driven ad generation system and key implementation steps for advertisers to optimize performance.
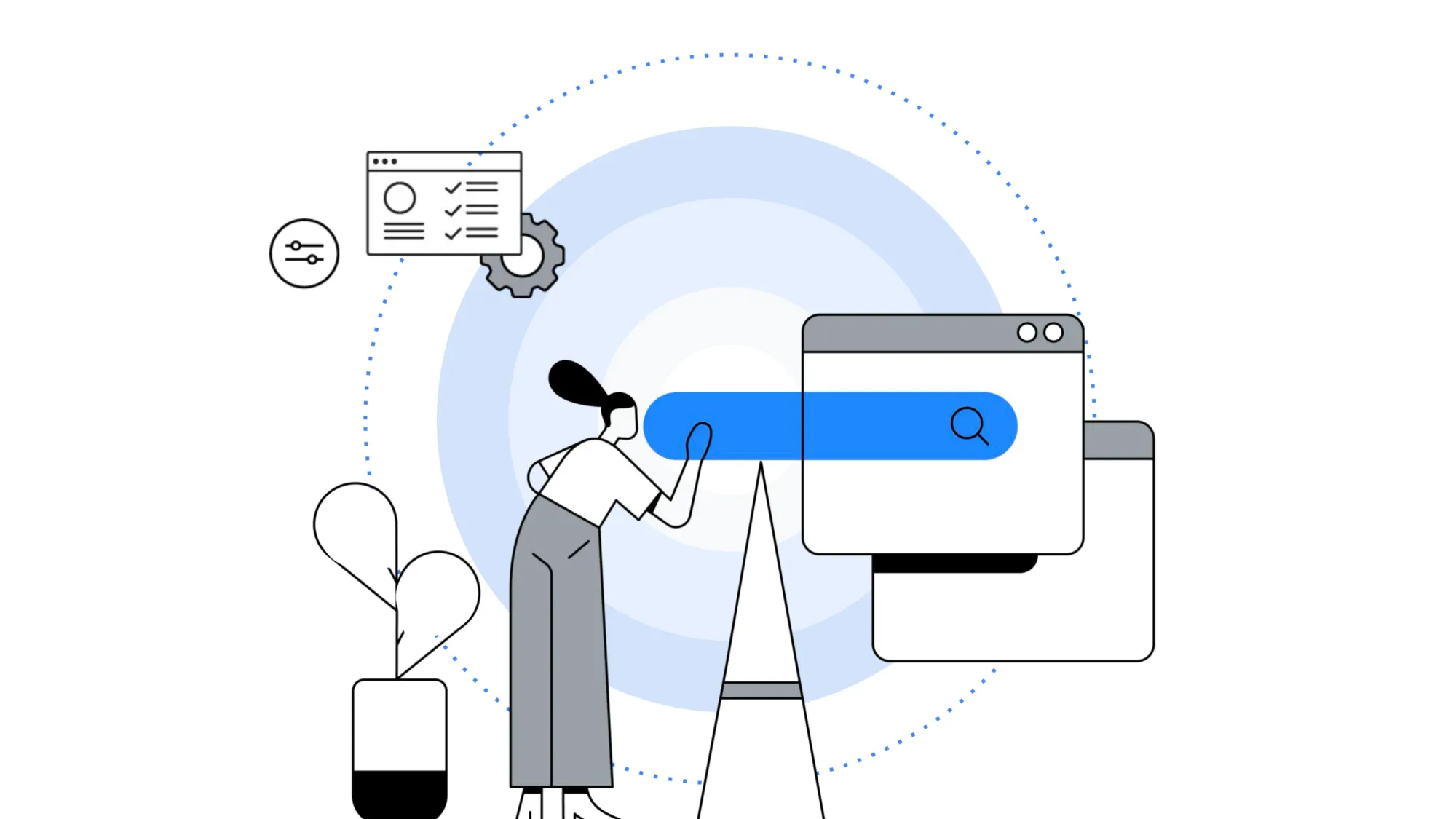
According to Google's technical documentation, responsive search ads employ artificial intelligence to dynamically generate advertisements through a sophisticated three-phase process. The system first analyzes search context, then assembles asset combinations, and finally evaluates and serves the most promising variations.
The AI begins by examining the context of each query and matching keywords, according to the technical specifications. This contextual analysis allows the system to understand user intent and match it with relevant ad content. The system then draws from the advertiser's provided assets - headlines and descriptions - to create combinations tailored to specific searches.
Internal Google data from January 2022 reveals that 15% of daily searches are entirely new, underscoring why static advertisements often fall short. The AI system addresses this challenge by continuously learning which asset combinations perform best for different query types.
For advertisers to leverage this AI effectively, several technical prerequisites must be met. The system requires substantial data volume to move beyond its initial learning phase. According to Google's documentation, individual assets need more than 500 impressions, while complete ads must accumulate over 2,000 impressions in the "Google Search: Top" segment across a 30-day period.
Asset diversity proves crucial for optimal AI performance. The documentation emphasizes that providing multiple variations of headlines and descriptions enables the system to test more combinations and identify the most effective matches for different search contexts.
Advertisers must focus on three core components when implementing responsive search ads:
- Asset Creation: The system offers automated assistance through asset suggestions, generating headlines and descriptions based on the provided Final URL. Additionally, an opt-in feature at the campaign level can automatically create tailored assets.
- Performance Monitoring: Google's Ad Strength measurement evaluates four technical aspects: headline quantity, headline uniqueness, keyword relevance, and description line uniqueness. These metrics were established through regression analyses measuring performance impact.
- AI-Ready Structure: According to the technical documentation, optimal performance requires integration with Smart Bidding and broad match capabilities, creating a comprehensive AI-powered advertising system.
The documentation highlights several important technical factors affecting performance. When advertisers use the pinning feature to ensure specific content always appears, this constrains the AI's ability to test combinations, potentially impacting effectiveness. The system also operates differently across varying query volumes, with higher volumes enabling more rapid learning and optimization.
Google's framework for evaluating AI-generated ads emphasizes business outcomes over traditional metrics. The technical documentation notes that changes in creative elements can affect impressions and clicks differently, requiring advertisers to focus on conversion metrics rather than click-through rates alone.
Implementation Best Practices
For optimal implementation, advertisers should:
- Maintain sufficient asset diversity for AI testing
- Ensure at least one responsive search ad per ad group achieves "Good" or "Excellent" Ad Strength
- Allow adequate impression volume for system learning
- Minimize pinned assets to maximize AI flexibility
- Focus on conversion metrics rather than intermediate measurements
According to Google's roadmap, the system continues to evolve across three technical areas: asset generation capabilities, relevance customization, and ad management tool improvements. This ongoing development suggests advertisers should prepare for increasing sophistication in how the AI matches advertising content with user intent.
The effectiveness of this AI-driven approach relies heavily on advertisers providing diverse, high-quality assets while allowing the system sufficient flexibility to optimize combinations. Success requires understanding both the technical requirements and the strategic implications of implementing responsive search ads within a broader digital advertising framework.