Why Predictive Audiences in GA4 are important after Google sunsets Similar Audiences
Predictive audiences in Google Analytics 4 (GA4) emerge as a potential replacement, leveraging machine learning for user behavior analysis and audience creation.
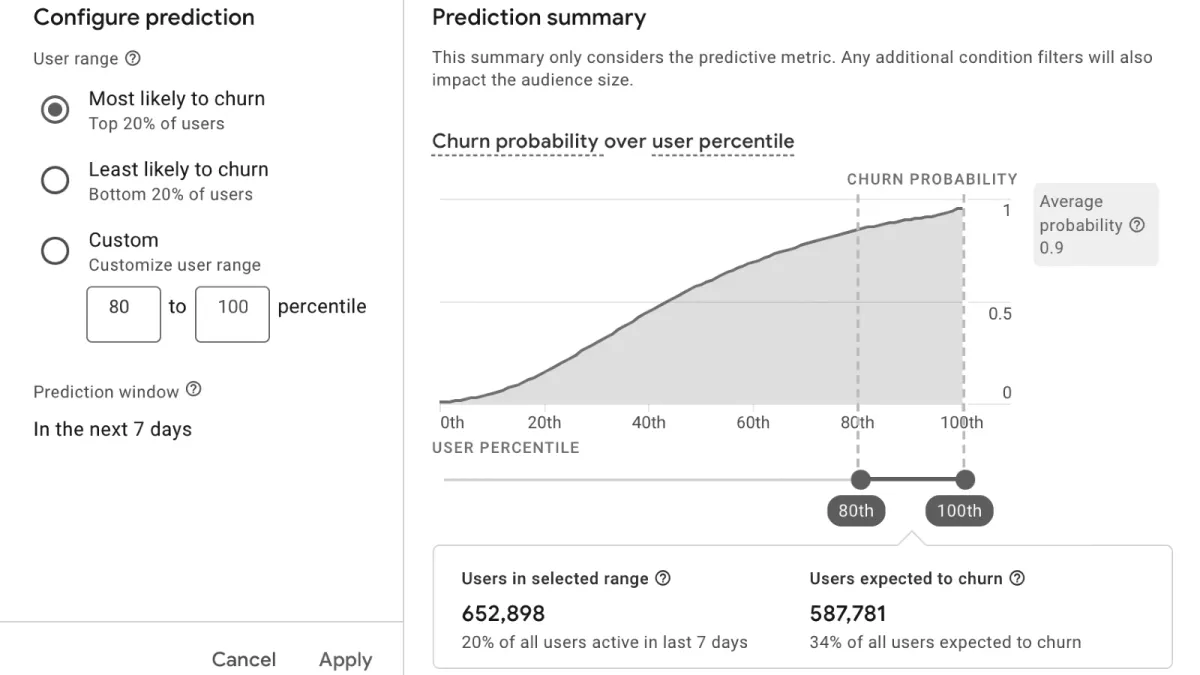
Google last year announced the sunsetting of Similar Audiences in Display & Video 360 (DV360) and Google Ads. This move away from reliance on third-party cookies for audience targeting highlights the need for alternative solutions for advertisers. Predictive audiences in Google Analytics 4 (GA4) emerge as a potential replacement, leveraging machine learning for user behavior analysis and audience creation.
A major factor driving the sunset of Similar Audiences is the phasing out of third-party cookies. These cookies track user behavior across different websites, allowing advertisers to build audiences based on browsing habits. However, growing privacy concerns and regulations are prompting stricter controls on third-party data collection. As a result, third-party cookies are becoming increasingly unreliable and less effective for audience targeting.
Similar Audiences vs. Predictive Audiences
Similar Audiences relied on historical user data and browsing behavior to identify new potential customers with similar characteristics to existing audiences. Predictive audiences in GA4, however, take a more future-oriented approach. They leverage machine learning models to analyze user behavior patterns and predict the likelihood of specific actions, such as making a purchase or abandoning the site.
Why Predictive Audiences are important
In the post-cookie era, marketers require new methods to reach target audiences effectively. Predictive audiences offer several advantages over Similar Audiences:
- Privacy-Centric: Predictive audiences rely on first-party data collected with user consent, addressing privacy concerns and adhering to evolving regulations.
- Forward-Looking: They predict future behavior instead of relying solely on past browsing activity, potentially leading to more relevant targeting.
- Actionable Insights: Predictive audiences go beyond identifying similar users; they highlight users likely to take specific actions, enabling more targeted marketing campaigns.
And while predictive audiences hold promise, their effectiveness depends on several factors:
- Data Quality: The accuracy of the underlying machine learning models relies heavily on the quality and quantity of data collected within GA4 properties.
- Model Development: Continuously refining and improving the machine learning models behind predictive audiences is crucial for maintaining their effectiveness.
- Integration with Advertising Platforms: Seamless integration of predictive audiences with advertising platforms like Google Ads will be essential for creating targeted ad campaigns.
Google Analytics 4 Predictive Audiences
Google Analytics 4 (GA4) offers several predefined templates for creating predictive audiences. Here's a breakdown of some key examples:
Purchase Behavior
- Likely 7-day purchasers: This audience targets users with a high predicted probability of making a purchase within the next seven days. This is valuable for focusing marketing efforts on users most likely to convert, potentially boosting sales.
- Likely first-time 7-day purchasers: This audience narrows down the focus to users predicted to make their first purchase within the next seven days. This can be particularly useful for acquiring new customers.
- Predicted 28-day top spenders: This audience identifies users forecasted to generate the highest revenue within the next 28 days. By targeting these high-value users, businesses can potentially maximize return on investment for marketing campaigns.
Churn Risk
- Likely 7-day churners: This audience includes users predicted to be unlikely to visit the website or app within the next seven days. Identifying users at risk of churn allows businesses to implement targeted re-engagement campaigns to win back potentially valuable customers.