Google cuts incrementality testing budget requirements to $5,000 minimum
Lower entry thresholds and Bayesian statistics enable smaller advertisers to measure campaign effectiveness accurately.
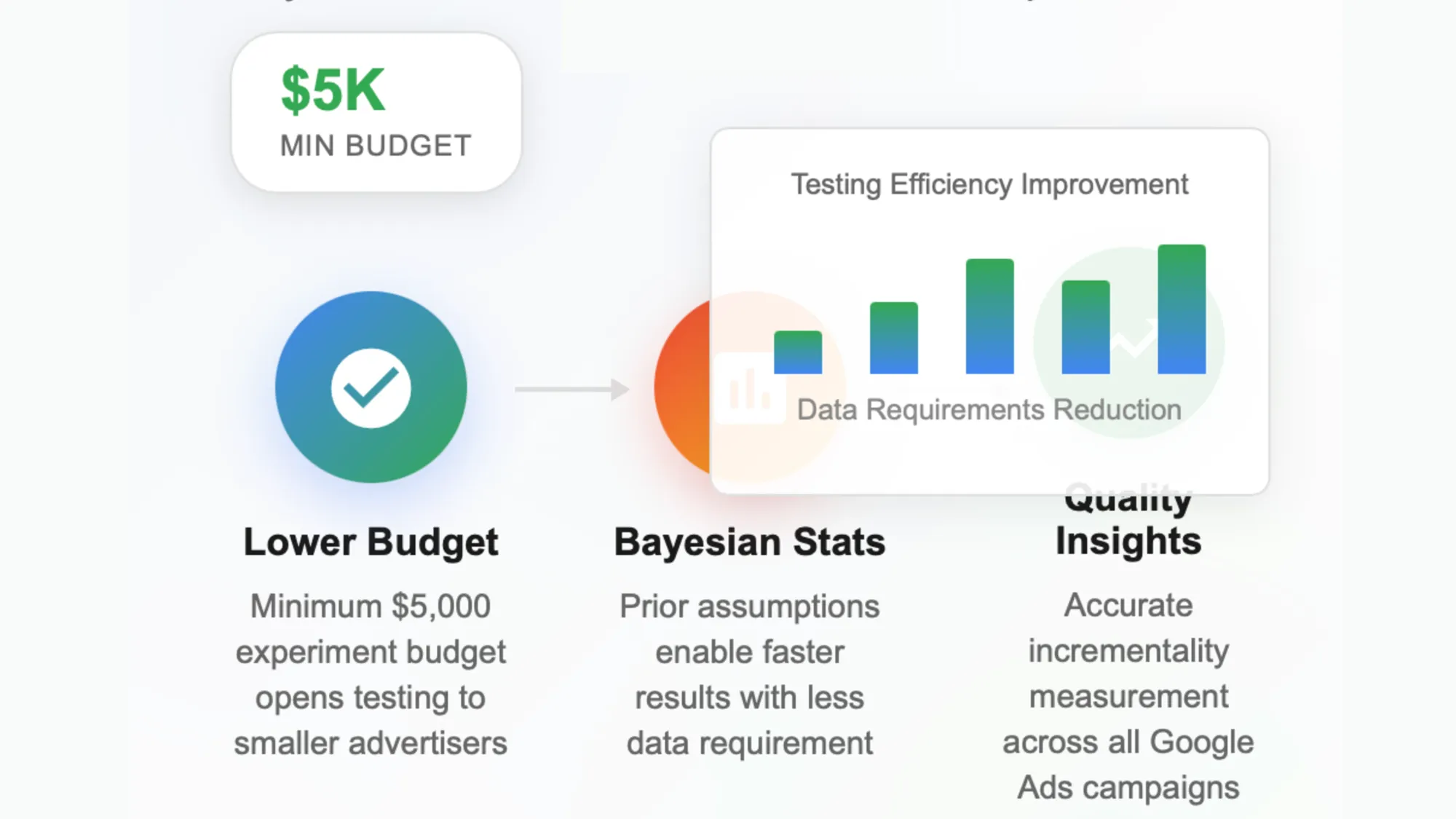
Google announced significant changes to its incrementality testing capabilities during Google Marketing Live 2025, held three days ago on May 22, 2025. The search giant introduced faster, more accessible incrementality testing that reduces the minimum experiment budget from previous higher thresholds to just $5,000. This development utilizes Bayesian methodology that relies on "prior assumptions" to deliver quality insights with substantially less data requirements.
Get the PPC Land newsletter ✉️ for more like this
The announcement represents a fundamental shift in how advertisers can measure the true impact of their advertising investments. Previously, incrementality testing required substantial budgets that placed this measurement technique beyond the reach of smaller advertisers. According to Ginny Marvin, Ads Product Liaison at Google, the new system is "available across all Google Ads campaigns" and enables advertisers to "launch an incrementality test with an experiment budget as low as $5K."
The core innovation lies in Google's implementation of Bayesian statistical methodology, which differs significantly from traditional frequentist approaches used in most marketing experiments. Bayesian statistics incorporates prior knowledge or assumptions about expected outcomes before analyzing new data. This approach allows for meaningful conclusions with smaller sample sizes compared to traditional methods that require extensive data collection periods.
According to statistical theory, Bayesian inference combines prior beliefs about parameters with observed data to produce posterior probability distributions. In the context of marketing measurement, this means advertisers can leverage existing knowledge about their campaigns, industry benchmarks, or historical performance to inform current experiments. The methodology updates these prior assumptions as new experimental data becomes available, creating a more efficient path to statistical significance.
The practical implication is profound. Traditional incrementality tests often required months of data collection and substantial spending to reach statistical confidence. The Bayesian approach can achieve similar confidence levels with less data by incorporating relevant prior information. This efficiency gain directly translates to lower budget requirements and faster decision-making for advertisers.
Google's system operates across all campaign types within its advertising platform. This comprehensive coverage includes Search campaigns, Display advertising, YouTube video campaigns, Shopping ads, and Performance Max campaigns. The universal availability ensures that advertisers can measure incrementality regardless of their primary advertising formats or strategies.
The technical architecture behind this capability requires sophisticated experimental design principles. Each test randomly assigns users to treatment and control groups, then measures the difference in conversion rates between groups exposed to advertising and those who were not. The Bayesian framework enhances this basic structure by incorporating prior knowledge about expected lift rates, seasonal patterns, or historical campaign performance.
According to measurement science principles, incrementality testing provides the most accurate method for determining advertising effectiveness. Unlike attribution models that assign credit based on user touchpoints, incrementality tests directly measure causation by comparing outcomes between users who saw advertisements and those who did not. This approach eliminates confounding variables that can skew attribution-based measurements.
The reduction to a $5,000 minimum budget threshold represents a dramatic democratization of incrementality testing. Previous systems typically required budgets in the tens of thousands of dollars to achieve statistical significance. This barrier effectively limited access to larger advertisers with substantial media investments.
Small and medium-sized businesses now gain access to measurement capabilities previously reserved for enterprise advertisers. A local retailer spending $20,000 monthly on Google Ads can allocate 25% of their budget to incrementality testing, generating actionable insights about their advertising effectiveness. This access enables more sophisticated media planning and budget optimization across business sizes.
The economic implications extend beyond individual advertisers. More widespread adoption of incrementality testing should improve overall advertising efficiency across Google's platform. When advertisers can accurately measure which campaigns drive incremental conversions, they naturally shift spending toward more effective strategies. This optimization creates a feedback loop that benefits both advertisers and the platform.
Google's announcement comes amid intensifying competition in marketing measurement and attribution. Meta has developed its own incrementality testing capabilities through Facebook's Conversion Lift studies. Amazon advertising offers similar measurement tools for its retail media platform. The reduced budget requirements position Google competitively against these alternatives while addressing a key barrier to adoption.
The timing also coincides with broader industry discussions about measurement accuracy and privacy-compliant attribution methods. As third-party cookie deprecation continues to impact traditional tracking methods, incrementality testing provides a privacy-friendly alternative that doesn't rely on individual user tracking. The methodology measures aggregate lift across user groups rather than tracking individual customer journeys.
According to marketing measurement experts, incrementality testing represents the gold standard for advertising effectiveness measurement. The methodology directly addresses questions about advertising causation that attribution models cannot definitively answer. By randomly assigning users to treatment and control groups, these tests isolate the true impact of advertising from other factors that might influence conversion rates.
Advertisers implementing the new incrementality testing capabilities must meet specific technical and strategic requirements. The system requires sufficient traffic volume to support meaningful statistical analysis, even with the enhanced Bayesian methodology. Campaigns with very low conversion volumes may still struggle to achieve statistical significance within reasonable timeframes.
The experimental design process involves several critical decisions. Advertisers must define primary metrics that align with business objectives, determine appropriate test durations, and establish expected effect sizes that justify the experimental investment. The Bayesian framework provides flexibility in these decisions by allowing for dynamic adjustment as data accumulates.
Google's implementation includes automated power analysis calculations that help advertisers understand the relationship between budget allocation, test duration, and expected statistical confidence. These tools guide optimal experimental design decisions based on specific campaign characteristics and business requirements.
The enhanced incrementality testing capabilities integrate with Google's broader measurement and analytics ecosystem. Results from incrementality experiments can inform attribution models, contribute to media mix modeling analyses, and calibrate other measurement methodologies. This integration creates a comprehensive measurement framework that addresses different analytical needs.
According to measurement best practices, incrementality testing works most effectively when combined with other measurement approaches. Attribution data provides tactical insights for campaign optimization, while incrementality tests validate strategic decisions about channel effectiveness and budget allocation. Media mix modeling incorporates incrementality findings to improve long-term planning accuracy.
The Bayesian methodology also enables integration with external data sources and measurement platforms. Advertisers can incorporate offline conversion data, customer lifetime value information, or brand awareness metrics as prior assumptions in their experimental design. This flexibility supports more sophisticated measurement strategies that align with complex business models.
The announcement signals broader shifts in marketing measurement philosophy and practice. Traditional approaches often relied on correlation-based analyses that struggled to establish causation. Incrementality testing directly addresses this limitation by using controlled experimental methods that isolate advertising effects from other factors.
For marketing organizations, the accessibility of incrementality testing changes strategic planning processes. Marketing teams can now validate assumptions about channel effectiveness, creative performance, and audience targeting with rigorous experimental evidence. This capability supports more confident budget allocation decisions and strategic pivots based on empirical data.
The reduced barriers to entry also enable more frequent testing cycles. Instead of conducting annual or quarterly incrementality studies, advertisers can implement ongoing testing programs that continuously refine their understanding of advertising effectiveness. This continuous learning approach aligns with agile marketing methodologies that emphasize rapid iteration and data-driven optimization.
Despite the advantages of Bayesian methodology, advertisers must understand important limitations and considerations. The quality of prior assumptions significantly impacts experimental outcomes. Poorly informed priors can bias results, while overly confident priors may not update appropriately based on new evidence. Successful implementation requires thoughtful consideration of relevant historical data and industry benchmarks.
The $5,000 minimum budget, while substantially lower than previous requirements, may still present challenges for very small advertisers or those testing niche campaigns with limited reach. The statistical requirements for meaningful incrementality measurement create practical floors below which experiments cannot produce reliable results.
According to statistical theory, Bayesian methods also require careful interpretation of results. Posterior probability distributions provide richer information than traditional confidence intervals, but this complexity can challenge marketers without strong statistical backgrounds. Google's implementation likely includes simplified reporting interfaces that translate statistical outputs into actionable business insights.
The introduction of accessible incrementality testing represents a significant development in digital advertising measurement. As more advertisers adopt these capabilities, the collective understanding of advertising effectiveness should improve across industries and campaign types. This enhanced measurement capability could drive more efficient advertising markets and better allocation of marketing resources.
For the marketing community, this development necessitates skill development in experimental design and statistical interpretation. Marketing teams must build capabilities in hypothesis formation, experimental planning, and results analysis to fully leverage these measurement tools. The democratization of advanced measurement techniques requires corresponding democratization of analytical skills.
The announcement also highlights the ongoing importance of measurement innovation in digital advertising. As privacy regulations and platform changes continue to impact traditional tracking methods, sophisticated measurement approaches like incrementality testing become increasingly valuable for maintaining advertising effectiveness insights.
Get the PPC Land newsletter ✉️ for more like this
Timeline
May 22, 2025: Google announces enhanced incrementality testing with $5,000 minimum budget and Bayesian methodology at Google Marketing Live 2025
January 29, 2025: Google releases Meridian open-source marketing mix modeling platform with Bayesian causal inference capabilities
January 5, 2025: Google publishes guide on building marketing experimentation capabilities, emphasizing systematic testing approaches
Related Coverage
- Google announces expansive AI advertising features at Marketing Live 2025 (May 23, 2025) - Comprehensive coverage of all Google Marketing Live announcements including incrementality testing improvements
- Google opens Meridian marketing mix model (January 29, 2025) - Details on Google's Bayesian-based measurement platform that complements the incrementality testing announcement
- Building experimentation muscle strengthens marketing effectiveness, says Google (January 5, 2025) - Strategic framework for implementing systematic marketing experimentation programs