IAB Tech Lab releases framework for privacy-first digital advertising without IDs
New industry guidance helps advertisers and publishers navigate the transition to ID-less solutions while maintaining advertising effectiveness and privacy standards.
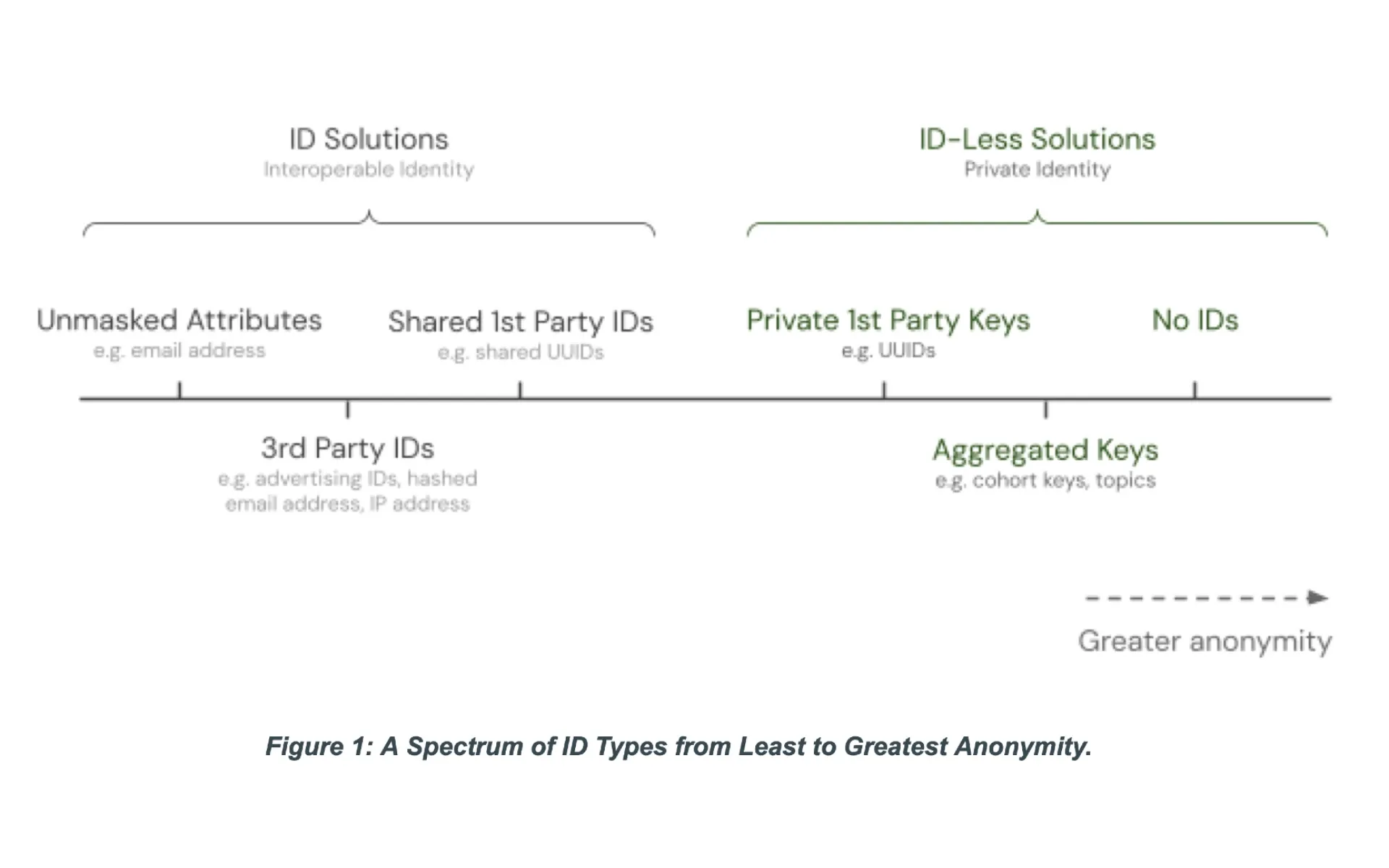
IAB Tech Lab last month announced the release of its comprehensive "ID-Less Solutions Guidance" document. The announcement, made on November 21, 2024, marks a significant step toward privacy-preserving digital advertising methodologies. This extensive framework, developed through collaborative efforts within the advertising technology industry, provides detailed technical specifications and implementation strategies for maintaining effective advertising capabilities without relying on traditional user identifiers such as third-party cookies and device IDs.
The guidance document enters a crucial public comment phase extending through December 19, 2024, allowing industry stakeholders to contribute their expertise and perspectives. This collaborative approach reflects IAB Tech Lab's commitment to developing practical, industry-wide solutions that address the complex challenges of modern digital advertising while prioritizing user privacy.
According to Shailley Singh, Executive Vice President and COO at IAB Tech Lab, the digital advertising industry is experiencing a transformative period that necessitates new approaches to addressability. "We are witnessing a fundamental shift in how digital advertising operates," Singh explained. "Traditional identifiers face increasing restrictions, profoundly altering how we conceptualize and implement advertising solutions. Our comprehensive guidance provides clear frameworks and actionable strategies for operating effectively in environments where individual IDs are no longer available or permitted."
The framework introduces several groundbreaking technical innovations designed to maintain advertising effectiveness while enhancing user privacy. At the heart of these innovations is the Private State Tokens system, an implementation of the Privacy Pass API developed by the Internet Engineering Task Force. This system enables robust validation of authentic user traffic without compromising individual privacy through tracking.
A significant component of the framework focuses on the implementation of the Shared Storage API, which enables critical advertising functions like frequency capping and creative sequencing while maintaining strict privacy standards. The guidance provides detailed technical specifications for leveraging this API effectively, including storage management strategies for maintaining campaign data, implementation patterns for cross-context frequency control, and privacy-preserving approaches to creative optimization.
The framework details innovative approaches to cross-context measurement without individual tracking, introducing several key methodologies. Through deterministic cohort-based measurement systems, advertisers can gain meaningful insights while preserving user privacy. These systems aggregate user behavior at the cohort level, enabling effective measurement without individual tracking. Complementing these deterministic methods, the framework describes probabilistic cohort approaches that can enhance targeting capabilities while maintaining privacy standards.
A significant portion of the guidance focuses on shifting processing to user devices, reducing the need for data sharing while maintaining advertising effectiveness. This includes detailed specifications for on-device auction implementations, local storage management, and privacy-preserving data processing protocols. The framework emphasizes secure communication protocols that protect user privacy while enabling essential advertising functions.
The framework addresses one of the most critical challenges in ID-less advertising: fraud detection. It introduces innovative approaches including device attestation systems that can effectively detect automated bot traffic without compromising user privacy. These systems leverage secure hardware capabilities and cryptographic protocols to validate authentic user interactions. Advanced statistical methods for identifying fraudulent patterns without relying on individual user tracking are thoroughly explained, including aggregate behavior analysis and pattern recognition algorithms.
Implementation considerations receive extensive attention in the framework, with comprehensive guidance on technical requirements and integration strategies. The documentation covers server-side components, client-side implementations, data storage requirements, and processing capabilities necessary for successful ID-less advertising solutions. Step-by-step guidance for integrating these solutions with existing advertising systems includes migration planning, legacy system compatibility considerations, and performance optimization protocols.
Privacy and security considerations permeate every aspect of the framework's technical specifications. The guidance details implementation strategies for various privacy-enhancing technologies, including differential privacy techniques, secure multi-party computation, and zero-knowledge proofs. Comprehensive strategies for implementing data minimization principles while maintaining advertising effectiveness address data collection limitations, storage restrictions, and retention policies.
The framework provides detailed specifications for implementing privacy-preserving aggregated reporting systems that offer meaningful insights while protecting user privacy. This includes methods for data aggregation, privacy threshold implementations, and statistical noise addition techniques that maintain reporting accuracy while preventing individual identification. Guidance on maintaining effective performance measurement in an ID-less environment covers alternative measurement methodologies, privacy-preserving attribution models, and conversion tracking approaches.
Technical challenges receive thorough treatment in the framework, acknowledging the increased complexity of ID-less solutions. The guidance addresses system architecture considerations, resource requirements, and scalability planning. Comprehensive strategies for managing privacy budgets effectively include methods for allocation, monitoring, and optimization, along with impact mitigation techniques.
The framework emphasizes the importance of industry collaboration in developing and maintaining effective ID-less solutions. Guidance on participating in and implementing industry standards ensures interoperability and consistent implementation across the advertising ecosystem. The documentation includes testing protocols and certification processes that help maintain quality and compatibility across different implementations.
Looking toward the future, the framework includes forward-looking guidance on emerging technologies and trends in ID-less advertising. This includes analysis of potential developments in privacy-preserving techniques, new measurement methodologies, and enhanced targeting capabilities. Guidance on maintaining flexibility and adaptability in implementations ensures solutions can evolve with technological advances and changing privacy requirements.
The framework provides practical recommendations for organizations implementing ID-less solutions, starting with comprehensive implementation strategies. This includes guidance on use case prioritization, resource allocation, timeline development, and risk management. Technical preparation requirements cover infrastructure assessment, capability evaluation, and testing protocols necessary for successful implementation.
Continuous monitoring and optimization receive significant attention in the framework. Comprehensive guidance on monitoring system performance includes identifying key metrics, measurement methodologies, and analysis techniques that maintain effectiveness while preserving privacy. Quality assurance requirements detail testing protocols, validation methods, and compliance verification processes essential for maintaining high-quality advertising solutions.
Chris Watts, Co-founder and CTO of NumberEight, emphasized the comprehensive nature of the guidance: "This framework illuminates the full spectrum of current and future tools available for key advertising use cases, helping businesses develop robust strategies amid growing signal loss. It provides practical solutions for maintaining advertising effectiveness while respecting user privacy."
The framework addresses the crucial area of campaign reporting and analytics in an ID-less environment, providing detailed specifications for privacy-preserving aggregated reporting systems. These systems offer meaningful insights while protecting user privacy through careful data aggregation, privacy thresholds, and statistical techniques that prevent individual identification while maintaining reporting accuracy.
The documentation also covers performance metrics and measurement methodologies adapted for ID-less environments. This includes guidance on alternative measurement approaches, privacy-preserving attribution models, and conversion tracking methods that maintain effectiveness without compromising privacy. The framework provides detailed information on calculating return on investment and other key performance indicators in privacy-preserving ways.
Implementation challenges receive thorough treatment, with the framework acknowledging and addressing technical complexities unique to ID-less solutions. This includes detailed guidance on managing system architecture, resource requirements, and performance optimization. The framework provides comprehensive strategies for managing privacy budgets effectively, including allocation methods, usage monitoring, and impact mitigation techniques.
Industry collaboration and standards development receive significant attention, with the framework emphasizing the importance of coordinated efforts in developing and maintaining effective ID-less solutions. Guidance on implementing industry standards ensures interoperability and consistent implementation across the advertising ecosystem, while testing protocols and certification processes help maintain quality and compatibility.
Looking toward the future, the framework includes forward-looking guidance on emerging technologies and trends in ID-less advertising. This includes analysis of potential developments in privacy-preserving techniques, new measurement methodologies, and enhanced targeting capabilities. Guidance on maintaining flexibility and adaptability ensures solutions can evolve with technological advances and changing privacy requirements.
As the industry continues to evolve, this framework provides a solid foundation for developing and implementing privacy-preserving advertising solutions. The public comment period through December 19, 2024, offers a crucial opportunity for industry stakeholders to contribute to this important initiative.
The comprehensive framework represents a crucial step forward in the evolution of digital advertising, providing the industry with clear guidance for maintaining effective advertising capabilities while respecting user privacy in an increasingly privacy-conscious digital ecosystem. Organizations can access the complete guidance document at https://iabtechlab.com/idless and submit feedback through the public comment period ending December 19, 2024.
Key Concepts from ID-Less Solutions Guidance
Understanding ID-Less Solutions
ID-Less solutions are methods for targeting ads and measuring advertising campaign performance without revealing personally identifiable information. These solutions focus on using contextual information, first-party data, and aggregated audience categories rather than individual user tracking. The key distinction is that ID-Less solutions prevent the ability to track specific individuals across different websites and contexts.
The Evolution of Digital Advertising
The advertising industry is undergoing a significant transformation in how it identifies and reaches audiences. This shift is driven by increasing privacy concerns, with browsers and platforms moving away from third-party cookies. The change represents a power shift between consumers, who are demanding more privacy controls, and advertisers who traditionally relied on detailed user tracking for targeting.
Benefits of ID-Less Approaches
ID-Less solutions offer several advantages, including greater coverage for publishers who can now monetize previously untrackable inventory, simpler compliance with privacy legislation, and improved consumer perception of online advertising. These solutions also enable personalized interactions without compromising individual privacy, allowing advertisers to maintain relevance while respecting user anonymity.
Challenges in Implementation
The transition to ID-Less solutions presents several challenges. Many technologies are still in their infancy, and some use cases lack proven solutions. Implementation costs can be substantial, and the current ecosystem remains heavily dependent on traditional identifiers. Additionally, measurement and attribution become more complex without individual-level tracking.
Attribution and Measurement
In ID-Less environments, attribution relies on alternative methods such as aggregated reporting, probabilistic cohorts, and media mix modeling. These approaches provide campaign insights while maintaining user privacy through techniques like differential privacy and delayed reporting. While less granular than traditional attribution, these methods can still effectively measure campaign performance.
Targeting and Prospecting
ID-Less targeting focuses on contextual data, cohort-based approaches, and seller-defined audiences. These methods allow advertisers to reach relevant audiences without individual tracking by considering factors like content affinity, browsing behavior patterns, and publisher-provided audience segments.
Fraud Detection
The transition to ID-Less advertising has led to innovations in fraud prevention, particularly through technologies like Private State Tokens. These solutions can actually provide stronger fraud detection capabilities than traditional cookie-based methods while maintaining user privacy. They enable verification of legitimate traffic without compromising user anonymity.
Future Outlook
The advertising industry is actively developing and refining ID-Less solutions to address various use cases. While some challenges remain, particularly around measurement and attribution, the industry is moving toward more privacy-preserving approaches that balance effective advertising with user privacy. This evolution suggests a future where privacy and personalization can coexist through innovative technical solutions.
Technical Implementation Considerations
ID-Less solutions often utilize advanced technologies like shared storage APIs, differential privacy, and on-device processing. These implementations require careful consideration of privacy budgets, reporting mechanisms, and data aggregation methods to ensure both functionality and privacy protection. The technical architecture focuses on keeping sensitive data processing either on the user's device or within strictly controlled environments.
Retargeting Mechanics in ID-Less Environment
In traditional retargeting, advertisers could track specific users across websites using persistent identifiers. The ID-Less approach revolutionizes this by using interest groups stored locally on the user's device. When a user visits a website, their browser can be assigned to custom cohorts that reside on their device. Instead of tracking individual users across sites, the device itself runs local auctions based on these stored interest groups. This maintains targeting capabilities while protecting user privacy by keeping sensitive data on the user's device.
Privacy Budget Concept
The privacy budget is a fascinating innovation in information theory applied to advertising. It quantifies how much information can be safely shared about user behavior without risking re-identification. When a user agent (like a browser) reaches its privacy budget limit, no additional data can be exported. This creates an intentional bias toward under-reporting rather than risking privacy violations. Think of it like a daily spending allowance - once you've used up your privacy "coins," you can't share more information until the budget refreshes.
Cross-Context Deterministic Cohorts
This approach offers a clever middle ground between individual tracking and complete anonymity. By storing observed events (like ad views or newsletter signups) in shared storage on the user's device, campaigns can measure outcomes by grouping events into cohorts. When integrated by both advertisers and publishers, it enables attribution capabilities without exposing individual user data. However, it's limited to single-device tracking and requires user consent in many jurisdictions.
Media Mix Modeling Evolution
The shift to ID-Less advertising has sparked renewed interest in Media Mix Modeling (MMM), a statistical approach traditionally used for TV and radio. Modern MMM uses advanced statistical models to attribute business results to campaign activities, typically validated through A/B tests. While this method provides privacy by design, it comes with longer measurement delays (6-8 weeks typically) and can only reliably attribute one advertising channel at a time, though workarounds are being developed.
Private State Tokens Innovation
Private State Tokens represent a breakthrough in fraud prevention without compromising privacy. When a user visits a token issuer's website and demonstrates genuine human behavior, tokens are stored in their browser. Other websites can later verify these tokens to confirm the user's legitimacy without knowing their identity. Unlike traditional fraud detection methods, this system prevents even the token issuer from correlating user behavior across different sites, creating a truly privacy-preserving trust mechanism.
Brand Lift Studies Adaptation
In the ID-Less world, brand lift studies have evolved to maintain effectiveness while respecting privacy. These studies now compare "exposed" and "control" groups at an aggregate level, measuring campaign impact through surveys that assess brand awareness and purchase intent. While this approach requires careful methodology to achieve sufficient scale, it provides scientific validation of campaign effectiveness without compromising individual privacy.
On-Device Frequency Capping
This represents a fundamental shift in how ad frequency is managed. Rather than relying on central servers to track impression counts, the user's device maintains a count of how many times specific creatives have been shown. When an ad is delivered, the device can signal whether frequency caps have been reached, maintaining user experience without exposing individual behavior patterns. This approach provides immediate frequency control but requires careful implementation to manage storage and campaign coordination across multiple publishers.
Seller-Defined Audiences Architecture
This framework enables publishers to leverage their first-party data in a privacy-preserving way. Publishers can insert audience labels into bid requests without sharing the underlying user data. While this approach offers scalability and encourages use of high-quality first-party data, it requires standardization of audience definitions and validation processes to ensure consistency across publishers. The industry is working on encryption and certification processes to improve signal quality while preventing data scraping.
Let me break down more fascinating concepts from the ID-Less Solutions document, explaining them in a way that builds deeper understanding.
The Privacy-First Transformation of Ad Technology
Think of the advertising world as undergoing a fundamental shift similar to how the automotive industry transformed from gas-powered to electric vehicles. Traditional advertising relied heavily on tracking individual users across the internet through cookies and identifiers - like following footprints in the sand. The new ID-Less approach is more like understanding patterns in crowd movement without following any specific person. This shift isn't just a technical change; it represents a complete reimagining of how digital advertising works while respecting user privacy.
Probabilistic Cohorts: The Art of Pattern Recognition
Imagine watching a crowd at a shopping mall without identifying any individual. You might notice patterns - groups of people who tend to visit certain stores at certain times. This is essentially how probabilistic cohorts work in ID-Less advertising. The system observes shared behaviors or characteristics among groups of users without knowing who they are individually. For example, it might recognize that people who read technology news in the morning tend to be interested in productivity software, without ever knowing their identities. This allows advertisers to reach relevant audiences while maintaining individual privacy.
The Evolution of Attribution Measurement
Traditional attribution was like following a single person's journey from seeing an ad to making a purchase. The new ID-Less attribution is more like understanding traffic patterns in a city. Instead of tracking individuals, it uses aggregate data and sophisticated modeling to understand how advertising affects overall behavior patterns. Think of it as similar to how city planners analyze traffic flow without needing to know which specific cars went where. This requires new statistical approaches and ways of thinking about success metrics.
On-Device Processing: Your Phone as a Privacy Guardian
One of the most innovative aspects of ID-Less solutions is the shift to on-device processing. Consider your smartphone or browser as a personal assistant who knows your preferences but never shares them directly. Instead of sending your data to advertisers, your device makes decisions about which ads might be relevant to you. It's like having a butler who knows your tastes but only tells visitors "yes" or "no" about whether you'd be interested in something, without explaining why. This represents a fundamental shift in where and how advertising decisions are made.
The Role of Differential Privacy
Differential privacy is like adding static to a radio signal - but in a very precise, mathematical way. When sharing aggregate data about groups of users, the system intentionally adds a carefully calculated amount of "noise" to the data. This noise makes it impossible to reverse-engineer information about individuals while maintaining the overall statistical usefulness of the data. It's a sophisticated balancing act between privacy and utility, backed by mathematical proofs that guarantee privacy protection.
The Interplay Between First-Party and Aggregated Data
In the ID-Less world, there's an important distinction between how first-party data (information a website collects directly from its users) and aggregated data are handled. Think of it like a restaurant that knows its own regular customers (first-party data) but only shares broad trends about dining patterns with other businesses (aggregated data). This creates a new dynamic where businesses must become better at understanding their direct relationships with customers while finding privacy-preserving ways to share insights with advertising partners.
Attention Metrics in Privacy-Preserving Advertising
The shift to ID-Less advertising has led to fascinating innovations in how we measure audience attention. Rather than tracking individual user behavior, new attention metrics focus on aggregate engagement patterns. Think of it like measuring the collective energy in a concert hall without identifying individual audience members. These metrics look at factors like active viewing time, content interaction, and engagement patterns at a group level. This gives advertisers meaningful insights about campaign effectiveness while preserving individual privacy.
Private Marketplace (PMP) Deals in the ID-Less Era
Private Marketplace deals have evolved to become more sophisticated in the ID-Less world. Imagine them as invitation-only auctions where publishers and advertisers agree on specific targeting criteria without needing to know individual user identities. What makes this particularly interesting is how it allows for precise targeting using first-party data while keeping that data private. For example, a publisher might know their audience includes technology enthusiasts, but instead of sharing user profiles, they simply confirm whether an ad opportunity matches the agreed-upon criteria.
Creative Sequencing Without Individual Tracking
One of the most innovative challenges being solved is how to tell sequential advertising stories without tracking individuals. Traditional advertising might track a person to show them a series of related ads in order. The ID-Less approach accomplishes this through clever use of on-device storage and local decision making. It's similar to how a book tells a story through chapters, but each reader decides when to move forward. The device keeps track of which parts of the story have been told locally, without sharing this information externally.
Automated Bot Detection in a Privacy-First World
The ID-Less ecosystem has developed sophisticated methods for detecting automated bot traffic without compromising user privacy. Instead of tracking individual behaviors, these systems look for patterns that indicate non-human activity at an aggregate level. It's similar to how security systems might detect suspicious patterns in crowd movement without identifying specific individuals. This approach actually proves more effective in many cases than traditional bot detection methods because it focuses on behavior patterns rather than identity markers.
The Evolution of Data Clean Rooms
While not strictly ID-Less, data clean rooms have evolved to complement ID-Less solutions. Think of them as neutral meeting grounds where different parties can analyze aggregate data without accessing individual records. What makes this particularly relevant is how they're being used to bridge the gap between ID-Based and ID-Less worlds during the transition period. They allow for sophisticated analysis while maintaining strict privacy controls.
Real-Time Bidding Adaptation
The real-time bidding process has undergone a fundamental transformation to accommodate ID-Less advertising. Instead of making decisions based on user profiles, the new system relies on contextual signals and aggregated insights. Imagine it as similar to how a newspaper might sell advertising space based on the content of articles and general readership patterns, rather than individual reader profiles. This requires new bidding algorithms that can make split-second decisions using privacy-preserving signals.
Privacy Budget Management Systems
The concept of privacy budgets has led to the development of sophisticated management systems that balance data utility with privacy protection. Think of it like an environmental impact quota system, but for privacy. These systems must make complex decisions about when and how to spend the privacy budget to maximize advertising effectiveness while ensuring user privacy isn't compromised. This includes predictive algorithms that help determine the most valuable times to use available privacy budget.
Let me take you deeper into the fascinating world of ID-Less advertising solutions, exploring the intricate mechanisms and innovative approaches that are reshaping digital advertising.
The Architecture of On-Device Auctions
Let's start with one of the most revolutionary concepts: on-device auctions. Imagine your device as a miniature marketplace, operating independently to protect your privacy. When you visit a website, instead of sending your data to external ad servers, your device runs its own auction locally. This process is particularly fascinating because it inverts the traditional advertising model.
Here's how it works: Advertisers provide bidding logic and creative assets that get stored on your device. When you visit a website, your device examines its stored "interest groups" (categories you've been assigned based on your local browsing) and conducts a real-time auction. The winning ad is selected based on relevance and bid price, all without exposing your personal data to external parties. Think of it like having a personal assistant who knows your preferences but only shares your final decisions, never the reasoning behind them.
The Mathematics of Privacy Budgets
Privacy budgets represent a sophisticated application of information theory to advertising. The concept is built on the mathematical principle that every piece of data shared about a user carries some risk of identification. Imagine you have 100 "privacy tokens" to spend each day. Each time your device shares information with an advertiser, it spends some of these tokens. The fascinating part is how the system calculates token costs.
Complex algorithms determine how much privacy budget to "spend" based on factors like:
- The granularity of the information being shared
- The uniqueness of the data points
- The potential for correlation with other shared data
- The time period over which data is aggregated
When your privacy budget is depleted, no more detailed information can be shared until the budget refreshes, ensuring your privacy remains protected even if multiple parties try to combine their data.
The Evolution of Attribution Science
Attribution in an ID-Less world has evolved into a sophisticated blend of statistics and behavioral science. Rather than tracking individual user journeys, new attribution models use advanced statistical techniques like:
- Multi-touch attribution modeling at the cohort level
- Incrementality testing using control groups
- Bayesian inference to estimate true campaign impact
- Time-decay modeling to understand the customer journey without individual tracking
Think of it like studying weather patterns instead of tracking individual raindrops. You can still understand the impact of your actions without needing to follow each particle.
The Role of Federated Learning
Federated learning represents one of the most promising frontiers in ID-Less advertising. This approach allows advertisers to improve their targeting models without ever seeing individual user data. Your device participates in model training by processing data locally and only sharing aggregate model improvements.
Imagine thousands of devices collaborating to improve an advertising model, each contributing their insights while keeping their data private. It's like a distributed research project where participants share their conclusions but never their raw data.
Real-Time Signal Processing
The transformation of real-time bidding signals in an ID-Less environment is particularly fascinating. Instead of relying on user IDs and behavioral profiles, the system now processes a rich array of contextual and aggregated signals in real-time, including:
- Content semantics and sentiment
- Time-based patterns
- Aggregate audience behaviors
- Publisher-provided cohort data
- On-device decision signals
The innovation lies in how these signals are processed and combined to make targeting decisions that are both effective and privacy-preserving.