Marketing Mix Modeling: A resurrected technique for today's marketers
Marketing Mix Modeling (MMM) sees a resurgence as a tool for marketers, with considerations for data privacy and limitations for smaller budgets.
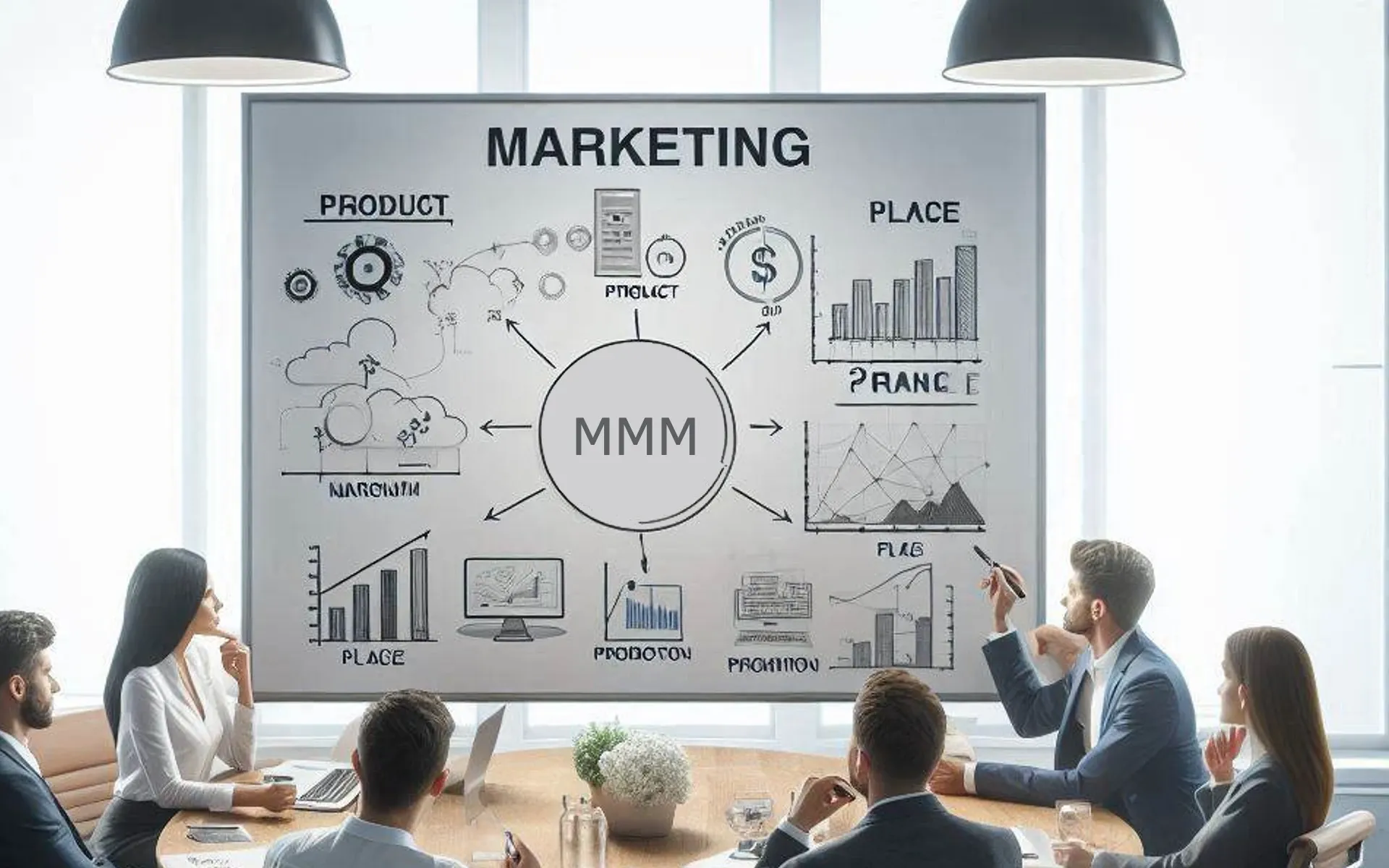
Marketing Mix Modeling (MMM) has re-emerged as a topic of discussion among marketing professionals. A recent LinkedIn post by Sebastian Hewing, a data analytics specialist, sparked debate around the technique's effectiveness in the current digital marketing landscape. MMM attempts to measure the impact of various marketing channels on a company's overall sales or revenue.
According to Hewing's post, he first encountered MMMs in 2007, a time when attribution modeling (identifying the touchpoints leading to a conversion) was still in its early stages. However, limitations in data collection often hindered the successful implementation of MMMs. The rise of directly measurable digital marketing channels like search engine advertising (SEA) and display advertising further diminished the perceived need for MMMs.
Hewing attributes the resurgence of MMM to growing data privacy concerns. As user privacy regulations tighten, marketers face challenges in attributing conversions directly to specific channels. MMMs can offer a holistic view of marketing effectiveness despite limitations in granular data.
Is MMM right for you?
Hewing cautions against a one-size-fits-all approach to MMMs. He proposes a framework based on a company's monthly marketing spend:
- Below €/$10,000: Focus on establishing strong data collection practices and proper channel/campaign naming conventions to lay the groundwork for future analytics efforts.
- €/$10,000 - €/$100,000: Prioritize basic attribution models like "last touch" or "first touch" while focusing on understanding user journeys and cross-device/platform attribution challenges. Consider attributing at least Gross Margin 1 (revenue minus cost of goods sold) instead of just revenue.
- €/$100,000 - €/$1,000,000: Refine operational cost calculations and start attributing Gross Margin 2 (gross margin minus marketing and sales costs) and Customer Lifetime Value (CLTV). Differentiate between new and existing customer acquisition. Explore implementing multi-touch attribution modeling. Begin preparing for MMM by experimenting with different media budgets to create data variance, a crucial factor for successful MMM implementation.
- €/$1,000,000+: Utilize MMM and attribution modeling together, along with incrementality testing (measuring the impact of additional marketing spend). Leverage MMM results for strategic decision-making and attribution modeling for tactical decisions. MMM can be used to estimate the impact of "unknown" marketing channels.
While Hewing's framework provides a starting point, it's important to consider additional factors. A recent article on PPC Land highlights Google's introduction of Meridian, an open-source MMM tool. This development suggests that the technology behind MMMs is evolving to address data privacy concerns.
Marketing Mix Modeling offers a way for businesses to assess the effectiveness of their marketing efforts, even in a privacy-focused environment. However, successful implementation requires careful consideration of factors like budget, data collection practices, and the availability of tools like Meridian. Marketers should carefully evaluate their needs and resources before deciding whether MMM is the right approach for their organization.
PPC Land is an international news publication headquartered in Frankfurt, Germany. PPC Land delivers daily articles brimming with the latest news for marketing professionals of all experience levels.
Subscribe to our newsletter for just $10/year and get marketing news delivered straight to your inbox. By subscribing, you are supporting PPC Land. You can also follow PPC Land on LinkedIn, Bluesky, Reddit, Mastodon, X, Facebook, and Google News.
Know more about us or contact us via info@ppc.land