Navigating the Hidden Risks of LLMs in Modern Marketing
An in-depth examination of privacy challenges posed by large language models and effective mitigation strategies for data protection compliance.
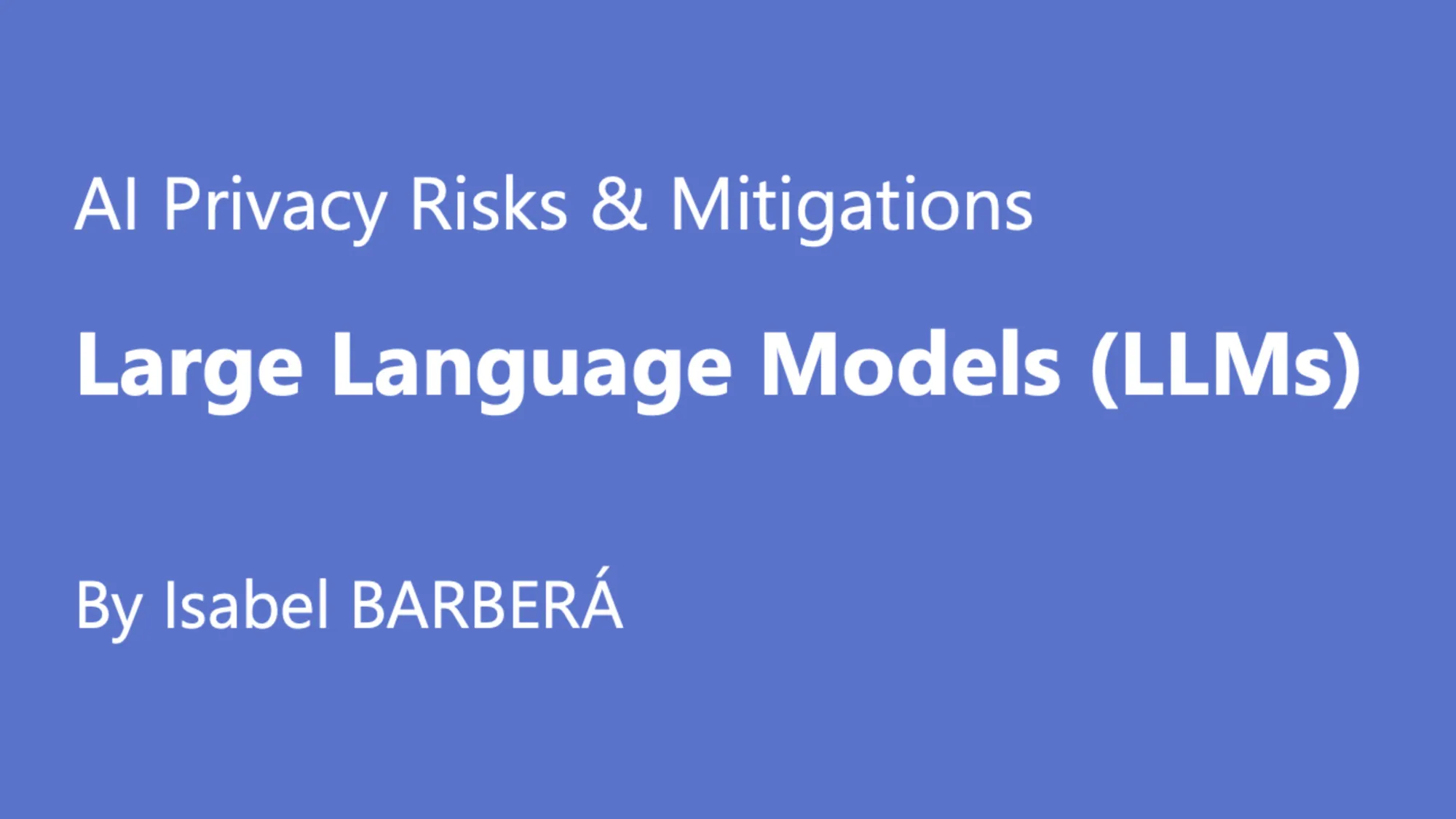
The European Data Protection Board (EDPB) published a landmark report on April 10, 2025, just four days ago, highlighting significant privacy risks associated with large language models. This comprehensive analysis, developed by expert Isabel Barberá through the Support Pool of Experts programme, offers critical insights for marketing professionals navigating the evolving artificial intelligence landscape.
Large language models (LLMs) represent a transformative advancement in artificial intelligence, trained on extensive datasets to process and generate human-like text. These powerful technologies have rapidly integrated into marketing workflows, from content creation to customer service, raising important questions about data protection and privacy compliance.
The report arrives at a critical juncture as organizations increasingly deploy LLM systems while facing heightened regulatory scrutiny. Marketing professionals must now balance innovation with robust privacy protections to maintain consumer trust and comply with evolving regulations. The EDPB's guidance provides a structured risk management methodology to systematically identify, assess, and mitigate privacy risks in LLM implementations.
Understanding LLM architecture and capabilities
Large language models function through sophisticated deep learning architectures, primarily using transformer models that process and generate text through attention mechanisms. These systems learn patterns from vast training datasets, enabling them to understand context and produce coherent, relevant responses.
Modern LLMs employ a multi-stage development process. During the training phase, models analyze extensive datasets to learn language patterns. The continuous improvement phase follows, where techniques like Supervised Fine-Tuning (SFT) and Reinforcement Learning with Human Feedback (RLHF) align the model's behavior with intended purposes. Finally, in the inference phase, the model generates outputs based on user inputs.
According to the report, "LLMs are predominantly accessible through several service models: LLM as a Service (via APIs), LLM 'off-the-shelf' (customizable pre-trained models), and self-developed LLM systems." Each model presents distinct implementation pathways with corresponding privacy implications.
Recent advances have introduced multimodal capabilities, enabling LLMs to process and generate content across different formats including text, images, and audio. This expansion of functionality increases both utility and potential privacy concerns as models handle diverse data types.
The report identifies a significant trend toward agentic AI systems—autonomous LLM-powered tools capable of planning and executing complex tasks with minimal human intervention. These systems introduce unique privacy challenges due to their expanded access to user data and their autonomous decision-making capabilities.
Critical privacy risks in LLM implementations
The EDPB report systematically categorizes eleven fundamental privacy risks that marketing professionals must address when implementing LLM solutions:
- Insufficient data protection: Inadequate safeguards may lead to unauthorized access, data breaches, or exposure of sensitive personal information. The risk increases with RAG (Retrieval-Augmented Generation) systems that connect to external knowledge bases.
An enterprise chatbot implemented without proper encryption protocols could expose customer conversations containing personal details to unauthorized parties. Similarly, poorly secured APIs might allow attackers to intercept sensitive data during transmission.
- Misclassification of training data as anonymous: Organizations may incorrectly assume training data is anonymous when it still contains identifiable information, leading to compliance failures and inadequate protection measures.
Dr. Kris Shrishak, who contributed to the EDPB's work on complex algorithms, emphasizes that "whenever information relating to identified or identifiable individuals whose personal data was used to train the model may be obtained from an AI model with means reasonably likely to be used, it may be concluded that such a model is not anonymous."
- Unlawful processing of personal data: Marketing organizations collecting data for LLM training without proper legal basis violate fundamental GDPR principles. The report notes that "web scraping as a method to collect data" requires careful legal assessment, particularly regarding legitimate interest balancing tests.
- Processing special categories of data: Marketing campaigns utilizing LLMs may inadvertently process sensitive data like health information or political opinions without meeting stringent GDPR exceptions. This creates significant compliance risks and potential harm to data subjects.
- Adverse impacts on fundamental rights: LLM outputs could contain biased or inaccurate information, potentially violating GDPR principles of accuracy and fairness. Marketing implementations must address these concerns to avoid misleading content that adversely affects individuals.
The report details how inaccurate outputs from marketing LLMs could impact consumers through misleading product recommendations or biased content, emphasizing the need for robust validation mechanisms.
- Lack of human intervention: Automated marketing decisions made by LLMs without human review may violate GDPR requirements for human oversight, particularly for decisions with significant effects on individuals.
- Failure to respect data subject rights: LLM systems may impede users from exercising their rights to access, rectification, or erasure of personal data. Marketing professionals must implement mechanisms to honor these requests effectively.
- Unlawful repurposing of data: Using collected data for purposes beyond those initially specified represents a critical risk. The report cautions against defining overly broad purposes like "developing and improving an AI system" instead of specific, well-defined objectives.
- Excessive data retention: Marketing LLMs may store personal data longer than necessary, violating storage limitation principles. Clearly defined retention policies must govern all collected information.
- Cross-border data transfers: Marketing operations using LLMs hosted in countries without adequate data protection standards create compliance risks under GDPR data transfer restrictions.
- Excessive data collection: Marketing implementations may process more personal data than necessary, breaching the data minimization principle. The report recommends regularly reviewing collection practices and eliminating unnecessary data.
Want to reach marketing professionals and decision-makers? Showcase your brand, tools, or services with our sponsored content opportunities.
Effective mitigation strategies for marketing implementations
The EDPB report outlines comprehensive mitigation approaches for each identified risk. For marketing professionals, several key strategies emerge:
Technical safeguards
Implement robust encryption for data in transit and at rest, secure APIs with authentication and access controls, and deploy privacy-enhancing technologies such as differential privacy to protect sensitive information.
"Defense in Depth approaches can be implemented by layering multiple risk mitigation measures to prevent single points of failure," the report advises. "This may include model-level protections, network security, user authentication, encryption, PETs, access control and continuous monitoring."
For marketing applications using RAG systems, implement careful model alignment to prevent unauthorized access and ensure strict security measures across all integrated data sources.
Governance measures
Establish clear data retention policies, conduct regular privacy audits, maintain detailed documentation of processing activities, and develop comprehensive incident response plans.
Marketing teams should maintain robust documentation to demonstrate compliance, including "details of DPIAs, advice or feedback from the DPO, information on technical measures to minimize identification risks during model design, and evidence of the model's theoretical resistance to re-identification techniques."
Transparency practices
Provide clear information to consumers about how their data is processed, implement user-friendly interfaces for exercising data rights, and communicate limitations of LLM systems.
The report recommends "explainability frameworks to analyze and understand how decisions are made," enabling marketing teams to identify potential sources of bias and enhance transparency for consumers.
Monitoring and evaluation
Regularly test for vulnerabilities, conduct adversarial assessments through red teaming, implement continuous monitoring systems, and periodically review risk management measures as technologies evolve.
Marketing applications should "regularly audit chatbot recommendations for fairness and transparency" and "clearly communicate to users how recommendations are generated" to maintain trust and compliance.
Practical risk assessment methodology
The EDPB report offers a structured risk assessment methodology specifically tailored for LLM implementations. This approach evaluates both the probability and severity of identified risks through quantifiable criteria.
For probability assessment, the framework considers factors such as frequency of use, exposure to high-risk scenarios, historical precedents, environmental factors, system robustness, data quality, and human oversight. These elements combine to produce a probability score on a four-level scale: Very High, High, Low, or Unlikely.
Severity assessment examines the nature of fundamental rights impacted, types of personal data involved, vulnerability of affected individuals, purpose of processing, scale of impact, contextual factors, reversibility of harm, duration of adverse effects, transparency mechanisms, and potential cascading impacts.
The framework employs a risk matrix that combines probability and severity scores to determine overall risk levels. Marketing professionals can use this structured approach to identify priorities for mitigation efforts, focusing resources on addressing the most significant risks first.
Case studies in marketing contexts
The report presents three practical use cases demonstrating risk assessment and mitigation in realistic scenarios. These examples provide valuable templates for marketing professionals implementing LLM systems:
- Customer service chatbot: A virtual assistant for responding to product queries, integrated with customer relationship management systems. The assessment identifies risks including potential data breaches, unauthorized access, and privacy violations. Mitigations include encryption, access controls, and transparent data usage policies.
- Student progress monitoring: An educational LLM system analyzing performance data to provide personalized recommendations. Though not directly marketing-focused, this example offers relevant insights for customer analytics applications, particularly regarding special categories of data and vulnerable individuals.
- Travel assistance agent: An AI assistant managing travel bookings and schedules, similar to marketing automation tools. The assessment highlights risks around third-party data sharing, cross-border transfers, and excessive data collection—concerns directly applicable to marketing automation implementations.
Implications for marketing professionals
The EDPB report signals increasing regulatory attention to LLM implementations in business contexts. Marketing professionals must now incorporate privacy considerations throughout the LLM lifecycle, from procurement and design to deployment and ongoing monitoring.
Effective risk management requires cross-functional collaboration between marketing, legal, IT security, and data protection teams. The methodology outlined by the EDPB provides a common framework to facilitate this cooperation.
The report emphasizes that risk assessment must be iterative and continuous, not a one-time exercise. Marketing teams should regularly review and update their risk assessments as technologies evolve, business processes change, or new threats emerge.
Importantly, the EDPB distinguishes between provider and deployer responsibilities under both the GDPR and AI Act frameworks. Marketing organizations deploying third-party LLMs maintain significant accountability for data protection compliance, even when using external services.
Timeline of key developments
- February 2025: Initial EDPB report on LLM privacy risks submitted by expert Isabel Barberá
- March 2025: Report updated with additional findings and recommendations
- April 10, 2025: Final report published through the EDPB's Support Pool of Experts programme
- Present: Organizations implementing the risk management methodology to enhance compliance
- Upcoming: Expected incorporation of findings into national supervisory authorities' enforcement approaches
The EDPB's comprehensive analysis arrives at a pivotal moment for marketing professionals leveraging artificial intelligence. By implementing the risk management methodology and mitigation strategies outlined in the report, organizations can harness the benefits of LLM technology while maintaining robust privacy protections and regulatory compliance.
As LLM technologies continue to evolve rapidly, this framework provides a durable approach to identifying and addressing emerging privacy risks. Marketing professionals who incorporate these practices will be better positioned to navigate the complex intersection of innovation and data protection in the AI-driven marketing landscape.