Research Paper: Google Improves Privacy-Preserving Ad Measurement
Google published a research paper on their blog post, describing how to significantly improve the accuracy of privacy-preserving ad measurement.
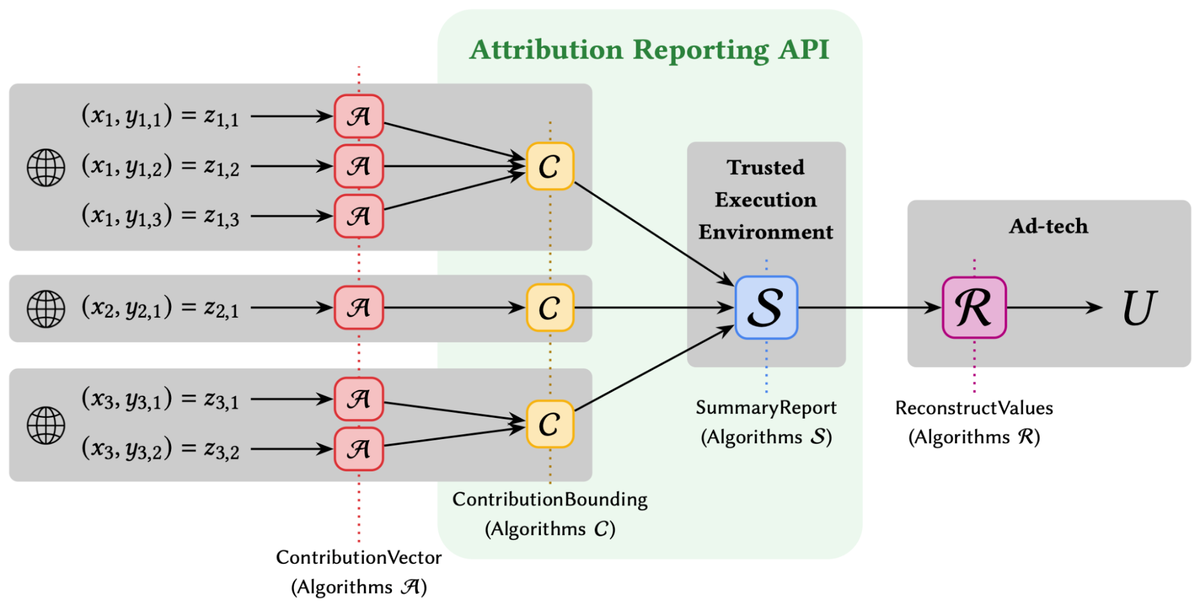
Google published a research paper on their blog post, describing how to significantly improve the accuracy of privacy-preserving ad measurement.
Researchers propose a new method for optimizing the configuration of Attribution Reporting API (ARA) parameters, which can substantially improve the utility of summary reports. The work shows that optimizing the parameters that can be set by the analyst can significantly improve the utility achieved by querying the API while satisfying the same privacy bounds.
ARA is a privacy-preserving API that is designed to help advertisers measure the effectiveness of their campaigns without compromising user privacy. ARA does this by encrypting individual user actions and collecting them in an aggregated summary report. This report can then be queried by advertisers to get estimates of the number and value of conversions attributed to their campaigns.
The task of configuring ARA parameters is important for maximizing the utility of the summary reports. In their paper, Google researchers describe a new method for optimizing these parameters. The method is based on a mathematical framework for modeling summary reports. They then formulate the problem of maximizing the utility of summary reports as an optimization problem and develop an algorithm for solving this problem.
The researchers evaluated their method using real and synthetic datasets, and they found that it significantly improved the utility of summary reports compared to baseline non-optimized summary reports.
This new method could have a significant impact on the way that advertisers measure the effectiveness of their campaigns. By improving the utility of ARA summary reports, advertisers can make better decisions about how to allocate their advertising budgets.
"We compare our optimization-based algorithm to a simple baseline approach. For each query, the baseline uses an equal contribution budget and a fixed quantile of the training data to choose the clipping threshold. Our algorithms produce substantially lower error than baselines on both real-world and synthetic datasets. Our optimization-based approach adapts to the privacy budget and data," wrote Hidayet Aksu, Software Engineer, and Adam Sealfon, Research Scientist, at Google.
This is a new development in the field of privacy-preserving ad measurement. It is likely to be adopted by advertisers who want to improve the accuracy of their campaign measurement.