Study finds ai language models degrade when trained on synthetic data
Research published in Nature reveals how ai models lose accuracy and capability when recursively trained on artificially generated content.
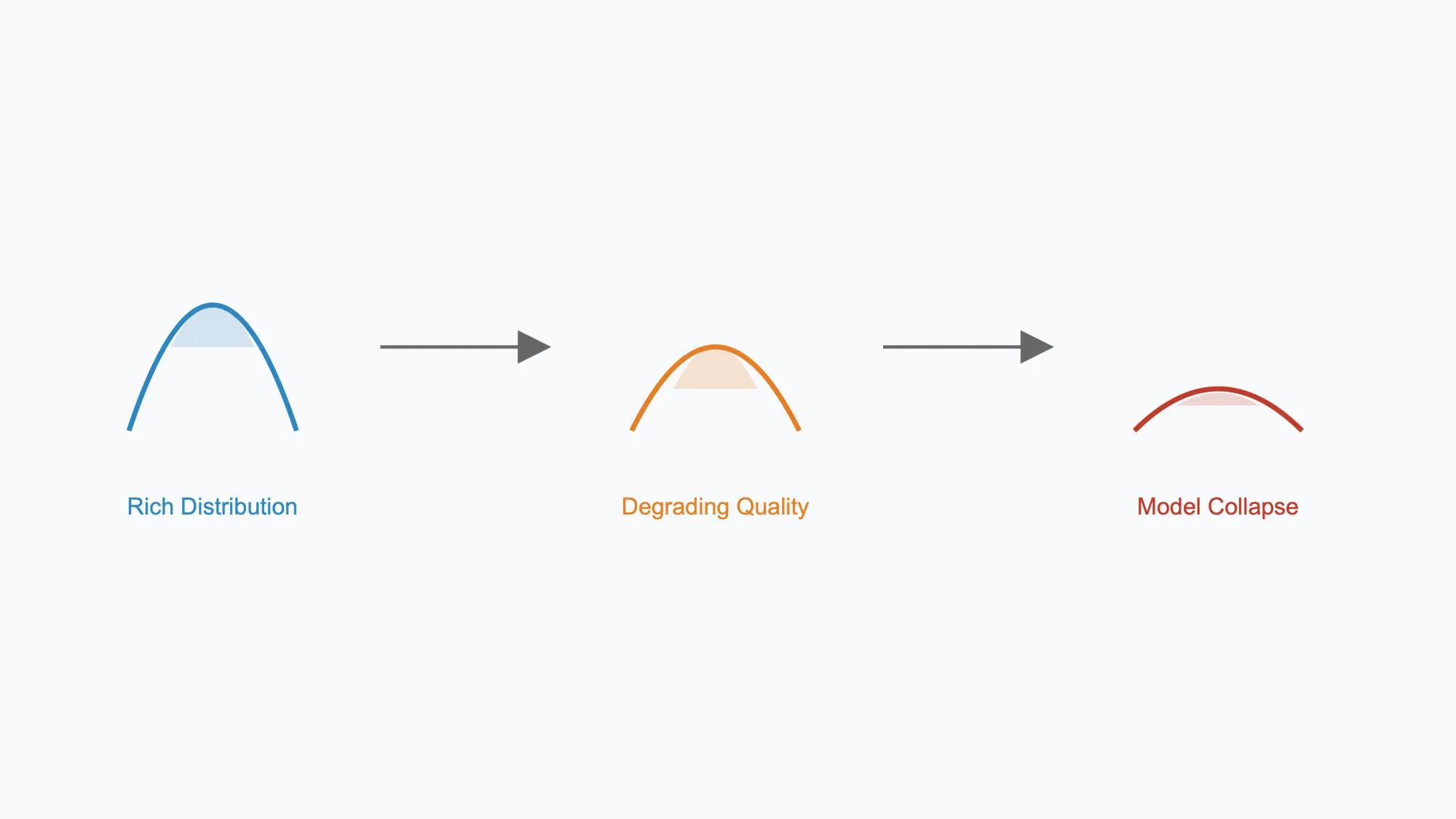
Large language models suffer irreversible degradation when trained repeatedly on artificial data, according to groundbreaking research published in Nature on July 24, 2024. This systematic study examines the impact of using machine-generated content to train successive generations of language models.
According to researchers from the University of Oxford and University of Cambridge, training artificial intelligence models on content created by other AI systems leads to what they term "model collapse" - a phenomenon where the models gradually lose their ability to capture nuanced aspects of language and complex patterns.
The study's lead authors, Ilia Shumailov and Zakhar Shumaylov, demonstrate that this degradation occurs across different types of AI architectures, including large language models (LLMs), variational autoencoders (VAEs), and Gaussian mixture models (GMMs). Their findings suggest fundamental limitations in using AI-generated data for training future AI systems.
Through extensive mathematical analysis and empirical testing, the researchers identified three main sources of error that compound across successive generations of models: statistical approximation error from finite sampling, functional expressivity limitations of neural networks, and errors arising from learning procedures.
The research team conducted experiments using Meta's OPT-125m language model trained on the wikitext2 dataset. After multiple generations of training on synthetic data, the models showed clear signs of degradation, with perplexity scores increasing from 34 to over 50 points in some cases. Even when preserving 10% of the original training data, model performance still declined noticeably across generations.
One striking example from the study shows how model outputs became increasingly detached from reality over successive generations. When prompted about historical architecture, the initial model produced relevant content about cathedrals and church design. By the ninth generation, the model generated nonsensical text about "jackrabbits" instead of architectural features.
The implications extend beyond academic interest. According to the researchers, this study raises critical questions about the future development of AI systems as synthetic content becomes more prevalent online. The findings suggest that access to high-quality human-generated data will become increasingly valuable for training robust AI models.
The research also highlights what the authors call a "first mover advantage" in AI development. As noted in the paper, "The need to distinguish data generated by LLMs from other data raises questions about the provenance of content that is crawled from the Internet: it is unclear how content generated by LLMs can be tracked at scale."
Professor Nicolas Papernot from the University of Toronto, a co-author of the study, emphasizes the importance of preserving access to original human-generated content. The research indicates that maintaining diverse, high-quality training data sources will be crucial for sustainable AI development.
The study's findings parallel historical challenges in web search, where content farms and low-quality synthetic content led major search engines to modify their algorithms. According to the researchers, Google previously had to downgrade automated content in search results, while DuckDuckGo removed content farm material entirely.
The mathematical framework developed by the researchers demonstrates that model collapse is not just an empirical observation but a theoretical inevitability under certain conditions. The team proved that even with optimal training conditions, models trained recursively on synthetic data will eventually converge to simplified, less capable versions of themselves.
This degradation manifests in two distinct phases: early model collapse, where the ability to handle rare or edge cases diminishes, and late model collapse, where the model's overall capabilities become severely restricted. The researchers found this pattern consistently across different model architectures and training regimes.
The study's publication in Nature marks a significant contribution to understanding the limitations of current AI training approaches. As synthetic content becomes more prevalent online, these findings suggest the need for new strategies to ensure the sustainable development of artificial intelligence systems.
Looking ahead, the researchers propose that the AI community may need to develop new approaches for tracking and managing synthetic content. They suggest that coordination between different organizations involved in AI development could help address these challenges, though implementing such systems at scale remains a significant technical challenge.